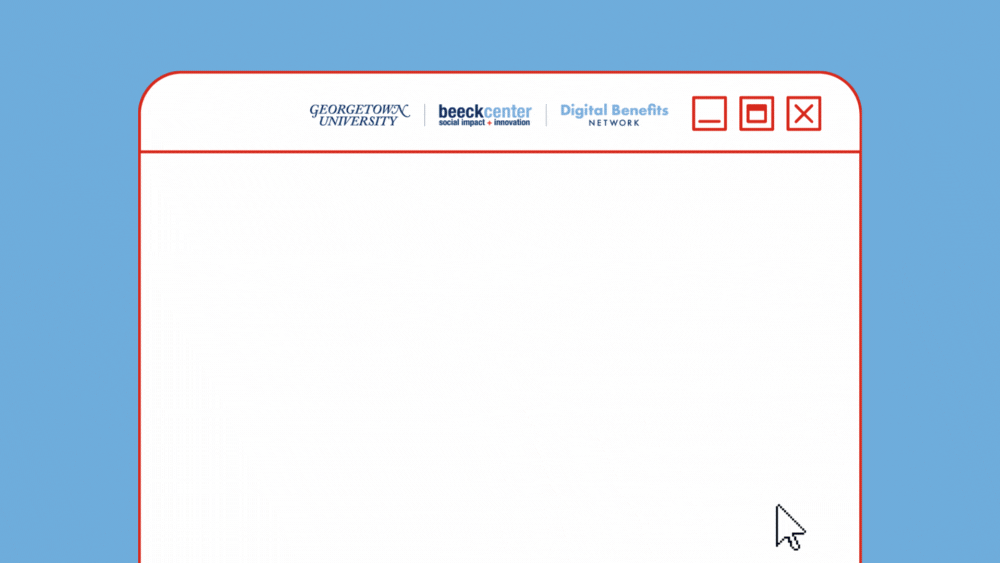
What the Digital Benefits Network is Reading on Automation
By Jason Yi, Student Analyst
Digital Benefits Network at the Beeck Center for Social Impact + Innovation at Georgetown University
September 2023
In 2023, conversations about artificial intelligence (AI) and automation became central in US media and news, raising debates about the potential benefits and risks of new technologies. In our reading and listening, the Digital Benefits Network (DBN) team has been paying attention to how different technologies are used, as well as approaches to automating processes and decision making in benefits delivery, rather than focusing on a specific technology.
It can be difficult to parse through the different terms used to describe the technology behind automated processes and systems—from artificial intelligence, to robotic process automation or algorithm-driven decision-making—and automated decision-making systems. It can also be challenging to sort through information about what a given technology can and cannot do today. Within the public benefits field, automation may describe uses of Robotic Process Automation (RPA) to streamline administrative tasks like assembling needed documents, or scheduling tasks. Generally RPA describes software that is process driven, meaning that they follow the rules specified by the user. However, other technologies can also be used to automate more complex and impactful components of benefits delivery, like fraud detection and eligibility determination, uses which have drawn scrutiny for many years. Automated systems may be touted for their potential: to ease case worker loads, process information quickly, and speed benefits access. However, the key word is potential. The impact of an uncaught bug, unforeseen biases, or an ethical oversight can be hugely consequential for end users, as seen when automated systems failed beneficiaries in Michigan, Arkansas, and elsewhere.
Following our facilitated sessions on Automation/AI at BenCon in June, we continue to gather resources and new information, and are sharing a list of several sources—from journalistic pieces, to reports and academic articles—we’ve found especially useful and interesting in our reading over the past few months. We hope that these resources are relevant for benefits practitioners getting oriented to key questions and definitions around automation, as well as anyone interested in automation or public benefits.
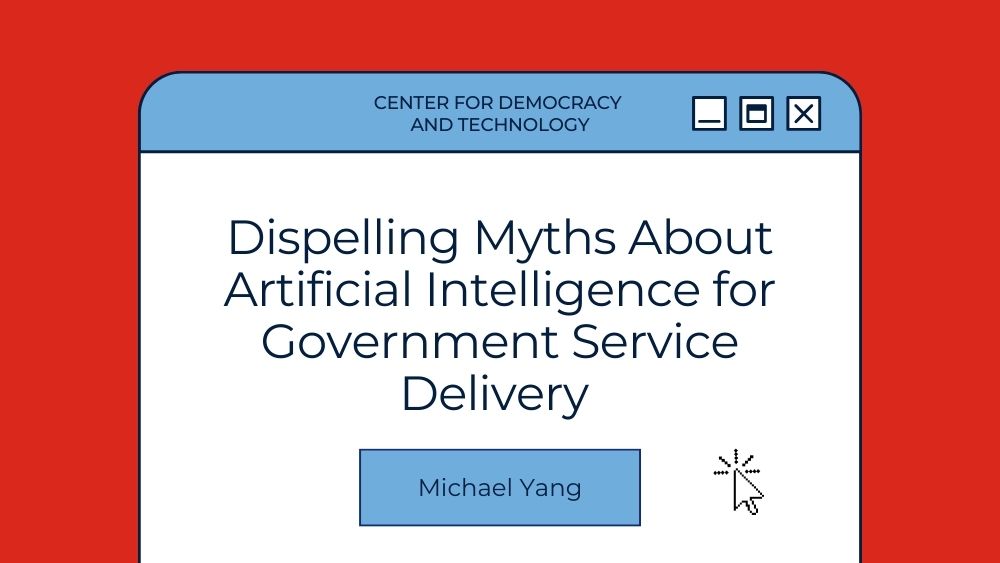
Dispelling Myths About Artificial Intelligence for Government Service Delivery
Center for Democracy and Technology | Michael Yang
In this brief, Michael Yang addresses common misconceptions about artificial intelligence (AI) and explains the current state of technology. Yang takes a no-frills approach to build understanding around four key points: there is not a shared definition of what AI is (and some technologies may be described as AI which are not, in fact, AI); some kinds of AI have made more technological progress than others; AI is not inherently objective or fair; and AI requires significant resources in order to function well. Directed at government practitioners, the brief suggests using specific terms to describe what a technology is doing, and outlines two key questions to evaluate whether an approach involves AI or not: (1) Does the application automatically make assessments, predictions, or judgments?; and (2) Does the application need to be “trained” or “tuned” on other data before it can be used? This concise brief offers a helpful level-set for thinking about AI in government contexts.
See also: In 2020, the Center for Democracy and Technology published a report on challenging benefits determinations from algorithm-driven decision-making systems. The report, which is directed at advocates, also includes a list of examples of algorithm-driven decision-making tools used for benefits determinations across states.
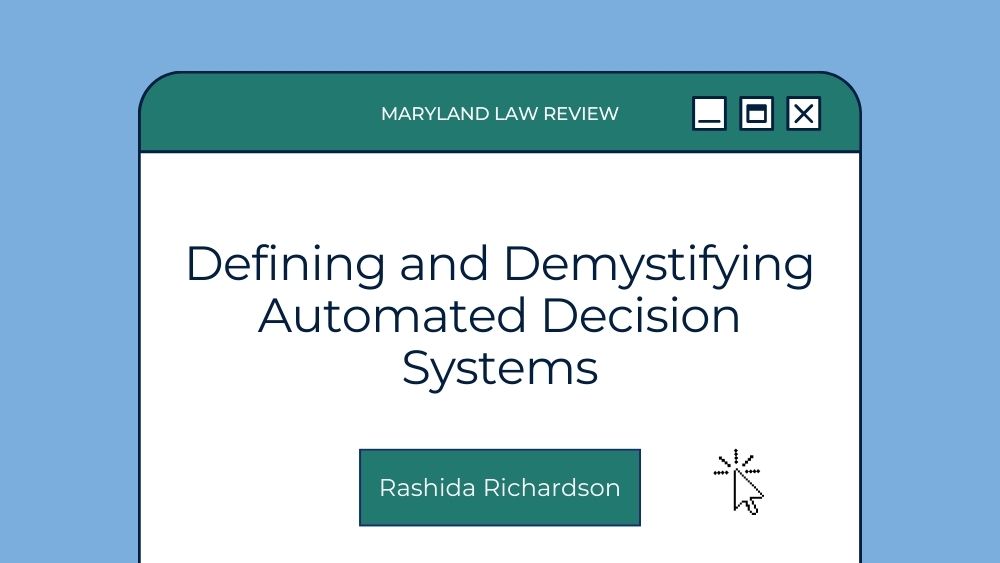
Defining and Demystifying Automated Decision Systems
Maryland Law Review | Rashida Richardson
Law and technology policy expert Rashida Richardson suggests that a lack of clear, shared definitions makes it harder for the public and policymakers to evaluate and regulate technical systems that may have significant impacts on communities and individuals by shaping access to benefits, opportunities, and liberty. Richardson notes that the term “algorithms” is often used to describe a range of technologies and approaches, only some of which fit under the category of artificial intelligence or automated decision systems. In the article, Richardson presents and evaluates a definition for automated decision systems, developed through workshops with interdisciplinary scholars and practitioners:
“Automated Decision System” is any tool, software, system, process, function, program, method, model, and/or formula designed with or using computation to automate, analyze, aid, augment, and/or replace government decisions, judgments, and/or policy implementation. Automated decision systems impact opportunities, access, liberties, safety, rights, needs, behavior, residence, and/or status by predicting, scoring, analyzing, classifying, demarcating, recommending, allocating, listing, ranking, tracking, mapping, optimizing, imputing, inferring, labeling, identifying, clustering, excluding, simulating, modeling, assessing, merging, processing, aggregating, and/or calculating.
See also: Richardson published a short guide in 2021, outlining best practices for government procurement of data-driven technologies, which includes a checklist of questions for practitioners to ask prior to procurement/implementation.
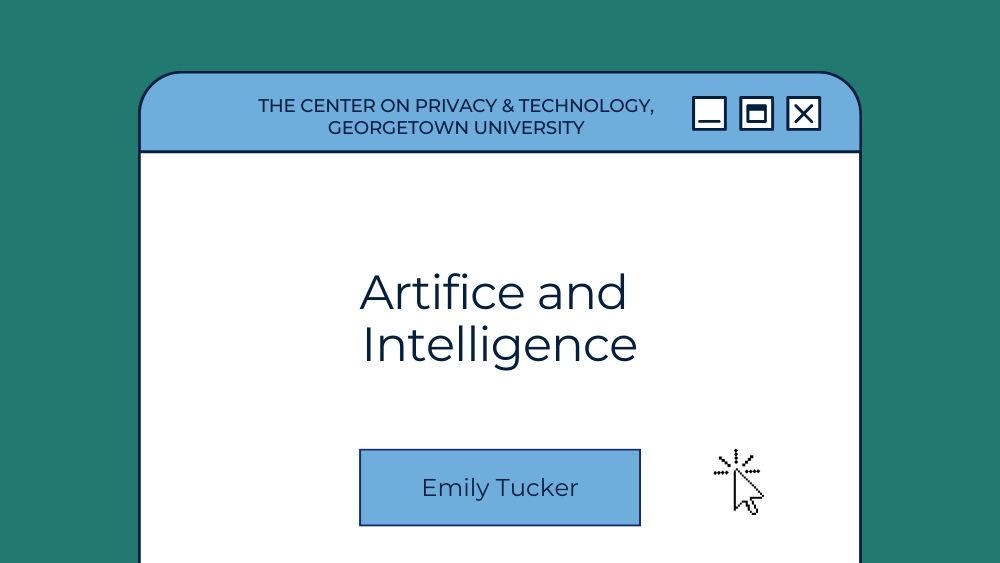
Artifice and Intelligence
The Center on Privacy & Technology, Georgetown University | Emily Tucker
In this piece, the Center on Privacy and Technology’s Executive Director Emily Tucker, outlines the Privacy Center’s decision to stop using the words “artificial intelligence,” “AI,” and “machine learning” in their work. As Tucker outlines, the public may not always have a clear sense of what AI means and may assume that AI systems are smarter than they are, based on connotations associated with terms like “artificial intelligence,” “AI,” and “machine learning.” To critically think about and evaluate these technologies Tucker explains that the Privacy Center plans to:
- Be as specific as possible about what a technology in question is and how it works;
- Identify any obstacles to their own understanding of a technology that result from failures of corporate or government transparency;
- Name the corporations responsible for creating and spreading the technological product; and
- Attribute agency to the human actors building and using the technology, never to the technology itself.
Used carefully and appropriately, terms like AI and machine learning have a place, but this piece from the Privacy Center raises important points around how to effectively talk about technology and agency.
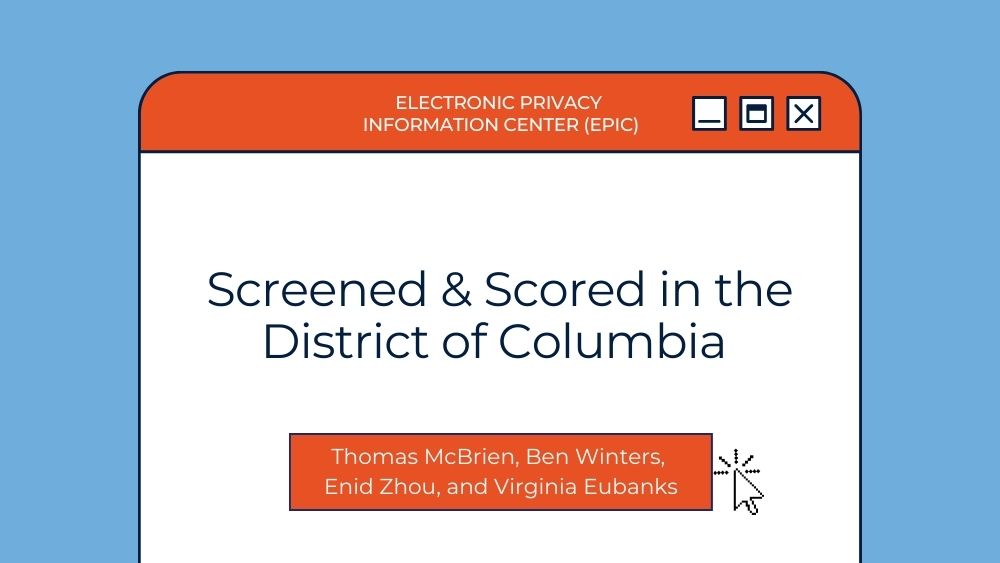
Screened & Scored in the District of Columbia
Electronic Privacy Information Center (EPIC) | Thomas McBrien, Ben Winters, Enid Zhou, and Virginia Eubanks
This report from EPIC, published in fall 2022, is a fantastic resource for understanding how automated decision-making systems are being used in one jurisdiction: Washington, D.C. Through Freedom of Information Acts (FOIA) requests, EPIC identified 29 Automated Decision-Making (ADM) systems in D.C. spread across 20 agencies. EPIC uses vignettes to humanize the impact of ADMs, and the resource carefully defines ADMs, categorizes current use cases of ADMs in D.C., and suggests actionable steps individuals, organizations, and policymakers can take to reduce the harms of ADMs. This is a comprehensive resource that explores the wider implications of ADMs by deeply exploring the use of ADMs in one place.
See also: Co-author Virginia Eubanks’ 2018 book, Automating Inequality: How High-Tech Tools Profile, Police, and Punish the Poor is a key text for examining the impacts of automated systems in government and benefits delivery specifically.
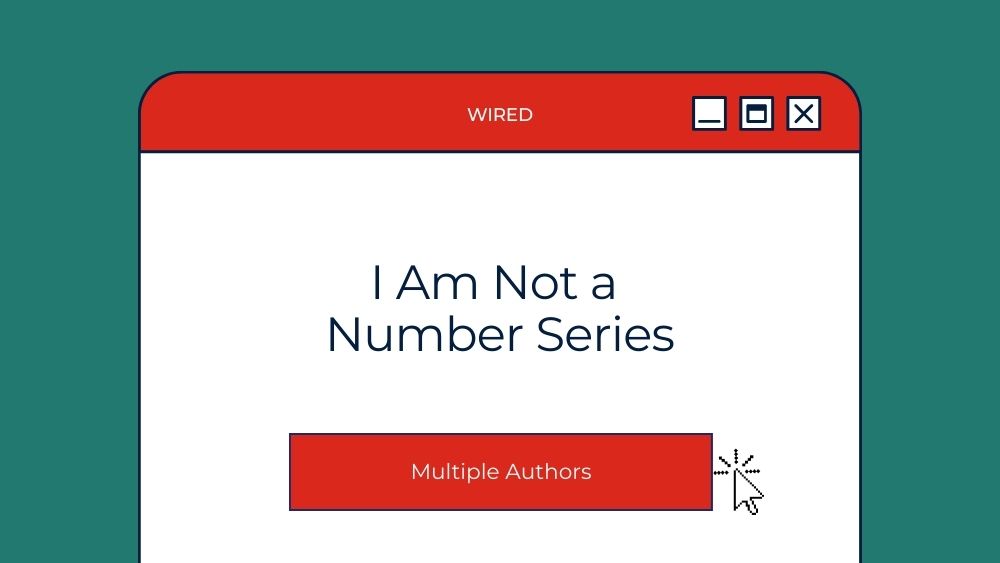
I am not a number Series
Wired | Multiple Authors
In early 2023, Wired magazine ran four pieces exploring the use of algorithms to identify fraud in public benefits. The series primarily focuses on cases from Europe. By sharing the stories of real people, this series underscores the burdens that biases in automated technologies, like algorithms used to detect fraud, may place on beneficiaries. The series also walks through how changes in inputs shape decisions returned by a risk scoring system.
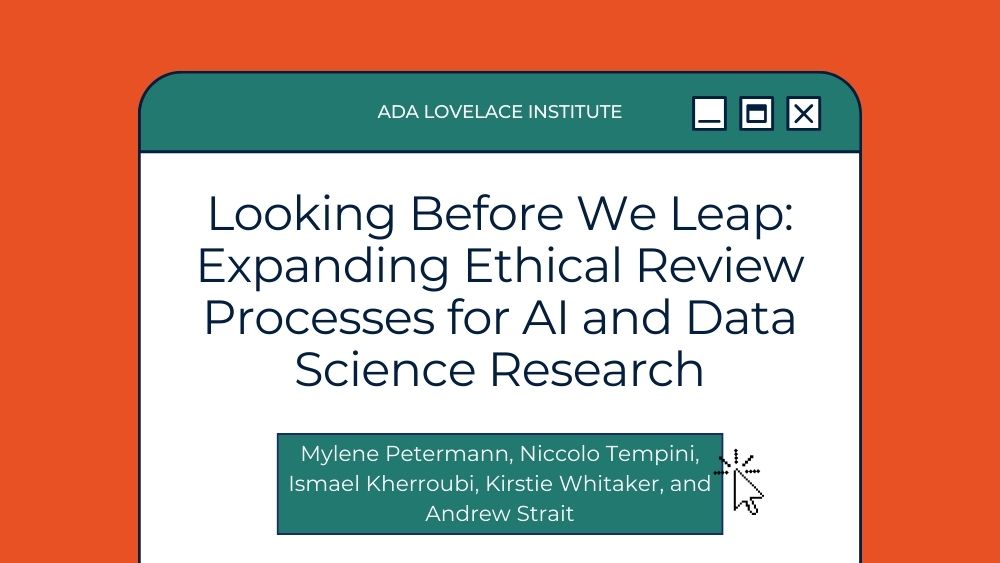
Looking before we leap: Exploring AI and data science ethics review process
Ada Lovelace Institute | Mylene Petermann, Niccolo Tempini, Ismael Kherroubi, Kirstie Whitaker, and Andrew Strait
In this report, the authors examine the current state of ethical review processes for AI and data science research. As they explain, the main way ethical risks are assessed and mitigated are through Research Ethics Committees (RECs). Effective research ethics practices can help minimize the downstream harms of AI; however, the authors suggest that current RECs are not equipped to effectively evaluate AI and data science research. They identify six challenges that RECs face when evaluating AI and data-science related research, including lack of resources, expertise, and training to appropriately address the risks that AI and data science pose, issues translating ethics from other fields, and—for corporate RECs—lack of transparency. The authors go on to provide specific recommendations to RECs, research institutions, and to funders, organizers, and other actors in the research ecosystem. This resource is helpful for understanding what it takes to responsibly develop AI and data science technologies. By understanding the research and development process, government practitioners can engage with in-house technical staff and vendors more critically to ensure responsible development practices and use of safeguards.
See also: The Ada Lovelace Institute also hosts other relevant resources on AI, including their 2021 report Algorithmic Accountability for the Public Sector, co-published with the AI Now Institute and the Open Government Partnership.
Keep Reading
The resources shared here only cover a portion of the conversation, and the DBN is continuing to collect and share resources on automation in government and, specifically, public benefits delivery, through a new Automation + AI topic page on the Digital Benefits Hub. That growing collection of resources features government documents, academic articles, and tools directed at government practitioners.
Additionally, federal government publications and resources also offer useful framing, definitions, and tools—from the White House Office of Science and Technology Policy’s Blueprint for an AI Bill of Rights, to the National Institute of Standards and Technology’s AI Risk Management Framework, to the AI Guide for Government from the General Services Administration’s Artificial Intelligence Center of Excellence. We are also following the work of many academic and independent organizations, including the AI Now Institute, DAIR (Distributed AI Research Institute), the Algorithmic Justice League, the Stanford University RegLab and the Stanford Institute for Human-Centered Artificial Intelligence.
We hope to continue sharing resources to introduce and distill key insights on this topic, to equip our community of public benefits practitioners to effectively evaluate new technologies with an ethics and equity lens.